“A good artificial intelligence system would anticipate the outbreak of a new pandemic in two or three weeks”
- Interview
- May 20
- 17 mins
Ramón López de Mántaras
Artificial intelligence (AI), a term coined in the late 1950s, is a common topic when talking about the future of work or the transformation of cities. The application possibilities for AI are endless, but they also pose ethical challenges to privacy and the right to privacy. López de Mántaras has been at the forefront of this field of knowledge since the mid-1970s. He is a pioneer in Spain and, in fact, alongside Luc Steels, he has been the driving force behind the Barcelona Declaration, a manifesto on AI that advocates the proper usage of this technology in Europe. We discuss why AI is so important today, the myths that surround it, the dangers it poses to privacy, and how it can help to plan smart, sustainable cities.
Ramón López de Mántaras is a Research Professor at the Spanish National Research Council (CSIC) and founder and former director of the Artificial Intelligence Research Institute (IIIA). He holds a PhD in Physics from the Paul Sabatier University in Toulouse (France), a Master of Science in Computer Engineering from the University of California-Berkeley, and a PhD in Computer Science from the Polytechnic University of Barcelona. Former editor-in-chief of AI Communications and an associate editor of Artificial Intelligence, the leading AI publication. He is an editorial board member of six international scientific journals. Author of almost three hundred articles on science, in 1982 he received the City of Barcelona Research Award and, in 2018, the Julio Rey Pastor National Research Award from the Spanish Government, among many other distinctions. His current research focuses on how to get computers and humanoid robots to learn from experience, and on artificial intelligence applied to music.
What explains the enthusiasm for and major media presence of artificial intelligence (AI) in recent years?
The story goes back to 2012. That year saw the publication of some remarkable findings as regards deep learning, one of the specialties of AI. A group of Canadian researchers developed a multi-layered artificial neural network that could recognise objects in an image database at enormous speed. ImageNet is a database comprising 14 million pictures of objects in different categories in which each image is tagged. The neural network was trained with 150,000 of these images, corresponding to a thousand categories of objects. Once trained, it was presented with images that belonged to these thousand categories but were different from the training images, and it recognised them with an accuracy of 85%.
Until then, the best algorithms had achieved 70% accuracy at most. In the years following the emergence of this neural network, other groups accomplished a 95% success rate. The Guardian published a news item that circled the globe: “Computers now better than humans at recognising and sorting images”, read the headline.
Beyond the gross exaggeration of the headline, the fact is that, in a short space of time, a giant leap had been made in the capacity of neural networks to generalise, make abstractions and capture the essence of the objects presented to them.
Does the secret of this AI milestone account for the large amount of data built into the system?
This is explained by the large amount of data in the ImageNet database and also that the algorithms were implemented in very powerful, high-performance machines with a high computational speed. An immense database and very powerful hardware explain why a two-decade-old algorithm achieved global prominence. The first to realise the economic possibilities of this breakthrough in AI were the Tech Giants (Facebook, Google, Baidu, etc.) and since then they have been deeply committed to deep learning. They were clear that they had the most valuable material – infinite data to train algorithms – and allocated significant resources to leading research work in this field. For example, Google immediately acquired DeepMind and turned a group of young entrepreneurs into billionaires. Today’s enthusiasm for AI has solid foundations.
Are this high point and the interest of Big Tech in AI good news for researchers? Is there room for research aimed at the common good?
The current interest in AI means that there are more resources for research, as it has become a priority area for research in the United States and the European Union. But we must try not to give false expectations and be cautious about AI’s capacity to solve complex social issues. The academic world is much less likely to have large amounts of data and access to huge computing power. We don’t have the resources or salaries that the Tech Giants offer to the brightest minds in universities.
However, the world of AI is very broad and there are still niches for the type of research that does not solely seek economic gain. Deep learning is one of the many branches of AI. There are many other areas of research that are of little interest to Google, Facebook, Baidu and other companies. Data constitute the fuel for deep learning, but there are also aspects with a knowledge focus. Therefore, we have a space in which to develop reasoning systems, to create hybrid knowledge-based and data-driven approaches, and to develop multi-agent systems that could prove useful in many areas.
The growing interest in AI has spread a series of myths that probably need to be clarified. I therefore propose an exercise in demystifying AI. First myth: Artificial intelligence works in a very similar way to the human brain. Is this true?
No, it’s false. Today, deep neural network systems, which some equate to the functioning of the human brain, actually model a tiny aspect of information processing in a brain. Today we know that when the neuron is activated and emits a signal, it is not only electrical in nature. In fact, there is a lot of information that the brain processes that is chemical in nature. Neuroscience has shown that glial cells, which are part of the nervous system, play a key role in the processing of brain information. And that isn’t integrated in any artificial neural model. Therefore, to claim that artificial neural networks are similar to the human brain is inaccurate. In fact, to date, AI has managed to develop simple, inaccurate mathematical models of the biological neuron.
So is the term “artificial intelligence” an exaggeration, in the sense that it is far from the capacity of human intelligence?
“AI has not always had the positive image it enjoys now, because of the expectations that some scientists and technologists generated.”
The name has undoubtedly proven a success. And it will continue among us. In fact, the term “artificial intelligence” has its history. The term “cybernetics”, coined by Norbert Wiener, was used in the early 1950s. But Wiener, considered the father of cybernetics, and another specialist, John McCarthy, didn’t have good chemistry on a personal level. In 1957, McCarthy organised a seminar at the University of Dartmouth, one of the oldest in the United States, and didn’t want to invite Wiener. That’s where the term “artificial intelligence” was coined as if it were a new area of knowledge.
The new denomination of cybernetics has taken root, but it has not always had the positive image it enjoys now, mainly because of the expectations that some scientists and technologists generated. In the mid-1990s, AI had bad press. In 1997, IBM’s Deep Blue software won a chess game against Garry Kasparov. When they asked IBM executives if Deep Blue was an example of AI, they denied it. They were afraid of scaring their customers. The term has become a buzzword today, but if we overstate the expectations, we could relive a new “winter” of AI.
Second myth: Intelligent machines learn on their own.
This is a highly exaggerated claim. Firstly, software has no purpose or intent. It does not learn by itself but rather is programmed. And this programming is very expensive – in terms of resources, qualified professionals, data collection, and definition of the specific system task – and it requires setting millions of parameters, and all of those tasks are performed by human programmers. Artificial intelligence is still a computer programme.
Third myth: Artificial intelligence can become 100% objective. It has no biases. Is that so?
This is clearly not the case. Such a system needs examples to be trained; the decisions it must make (sorting an image, driving an autonomous vehicle) are learned from human-generated data. This is the case with a machine that performs medical diagnoses: doctors generate data about a disease that predetermines the results. The reality is that the data can be skewed. For example, this applies to the system implemented in the United States that provided advice on whether or not to grant parole to inmates based on their likelihood of recidivism. Behind it was an algorithm that was shown to discriminate against African Americans. Why? Because the training data in the system had biases that discriminated against this group. An African-American prisoner who disagreed with the decision appealed and requested he be given the arguments behind the denial of parole and they were unable to do so. The judge, to save himself work, didn’t go beyond the machine’s “advice”. The prisoner won the appeal and the whole system was called into question. I’m not against these systems to help make decisions, but first we need to analyse the possible biases, and determine what they are and whether they can be corrected. These systems cannot be hastily put on the market; they should previously go through some kind of VTI for algorithms.
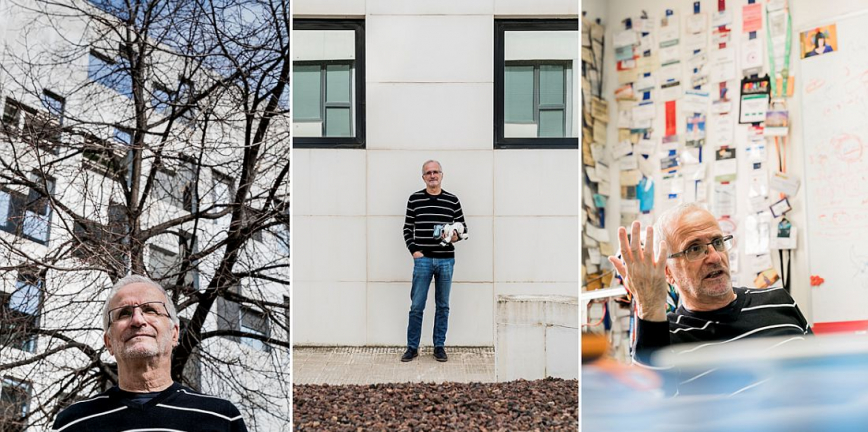
Fourth myth: Artificial intelligence only replaces humans in the simplest tasks. Is it an idea that needs to be nuanced?
It’s not quite like that. There are simple tasks that can be performed by a given machine that call for manual skills unattainable by a device. For example, some jobs performed by an electrician or many household chores (cleaning or putting on the laundry). These are tasks that seem simple but require a great deal of dexterity.
There are also intellectual jobs that a machine could do. For example, the work of a lawyer when preparing a legal defence through the consultation of databases or the writing of a standard newspaper article... Can an AI machine do this?
It can. And it can even imitate a certain journalistic style, as long as it has enough texts to build patterns. A machine can quickly analyse an extensive body of data. But we must be careful: more data does not mean more intelligence. The key lies in the quality of the data. Making good or bad decisions does not only depend on the quantity but also and mainly on the quality of the data. What inconsistent data, which incorporate noise, do is make decisions worse. Artificial intelligence can therefore replace routine work, whether manual or intellectual, more than simple intelligence. However, if we are faced with unforeseen, uncertain, undetermined situations that do not respond to patterns, machines have little to do. In contrast, humans do know how to respond, better or worse, to unforeseen situations.
The last myth: People who work in the primary sector, agriculture or livestock breeding and claim that artificial intelligence does not affect them, are they wrong?
Artificial intelligence is very horizontal. Take an agricultural entrepreneur who has to manage a great deal of information and uses drones to control the harvest or take market data into account, or even climate variables to make better decisions. Not even the art world escapes AI.
Does that mean there are machines that are programmed to be creative?
They can be, to some extent. Creativity is not a dual activity, but it has degrees. Obviously today’s machines cannot break rules and invent a new musical or pictorial style like the Cubists or the inventors of atonal music did. Instead, from the exhaustive analysis of a musical or pictorial style, deep learning systems can extract patterns from the style of a painter like Rembrandt, a composer like Bach, or any artist. Once the machine has captured the model, it is able to generate new paintings or compositions. This has already been done. Can this be called creativity? Many people argue that this isn’t creative, but they are really original and valuable works. I think that, at the very least, they undeniably have a certain artistic value.
Developing algorithms has a creative aspect, but at the same time sparks misgivings for the biases they can include. In fact, you are one of the promoters of the Barcelona Declaration, which establishes the ethical playing field when it comes to developing algorithms. What should the ethical principles be regarding the development of artificial intelligence?
First, caution. For example, not rolling out applications before determining the biases they have. Second, accountability. Systems cannot be black boxes and the processes established to justify decisions should be transparent. And, above all, not to forget the human being, that is, not to replace a machine with a person based solely on profitability criteria. In addition, it has been shown that better decisions arise from human-machine collaboration than from just a machine. We have seen this in the field of medicine. However, in other areas, it makes perfect sense to make a radical replacement. This is the case of the level 5 autonomous car which, when it is mainstream – that will still take time – will save thousands of lives on the road. In this case, the benefit for society as a whole is undisputed.
Can it be determined whether algorithms are free of bias?
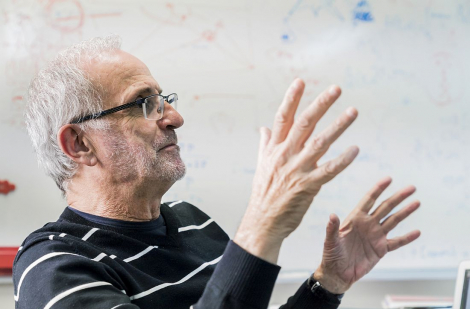
It’s not always easy, but it can be done. It’s best to verify and test them before deploying them. Bias can be present not only in the data but also in the algorithm itself. Algorithm programmers may or may not have entered the bias intentionally when writing the code that programmes the algorithm. If the code is complicated, it isn’t easy to decipher. Furthermore, when the algorithm is very complex and requires thousands or even millions of lines of code, programming is the work of a team, often comprising several members, that integrates, step by step, the various components of the programme created jointly.
Do you think it is feasible to agree on a global ethic for artificial intelligence?
I don’t think so. Except in very basic matters, we cannot speak of a universal ethic across countries and cultures. If I had the solution to the problem of ethics in AI, it would be the cover of Time magazine. There are interesting proposals, such as the one made by Professor Stuart Russell from the University of California at Berkeley in his book Human Compatible, yet his proposal to ensure control of future machines, however intelligent they may be, touches on science fiction.
Finally, the responsibility lies with designers and programmers, as well as with users. This is where we need to focus and control. Moreover, more ethics content should be incorporated into science and engineering studies. In fact, ethics will be featured in the new artificial intelligence degrees Catalan universities are preparing. They will be technical degrees in which humanistic and ethical aspects will be fundamental. We’re late to do so, but we’re finally threading the needle.
Do you think that artificial intelligence and robotics are essential if we want to preserve the welfare state?
Artificial intelligence has extraordinary potential to improve the well-being of our societies. In fact, many applications already help doctors make decisions. Whether we go this route or not will depend on political will and investing the necessary resources.
And in the case of Covid-19, what could have been done with AI that was not done?
“We still have time for AI to be useful in diagnosing cases and treatments, and especially in helping and speeding up the development of a vaccine.”
AI, contrary to what we have read in some news items, didn’t perform a better detection than that of human specialists. But we still have time for it to be useful in diagnosing cases and treatments, and especially in helping and speeding up the development of a vaccine. In fact, there are many groups around the world working with AI support.
In the future, in the event of another pandemic, it could be detected and anticipated that something strange is happening. If a lot of high-quality data are available – comments from reliable sources on social media and real information from medical centres – patterns that do not match normal patterns of behaviour could be detected. This would allow early detection of incidents such as Covid-19. The next step would be for experts to interpret the data provided by the software and confirm abnormal behaviour and its severity. Weeks could even be saved before an outbreak.
But this massive monitoring has a dark side: the threat to privacy.
That would be the danger. In principle, information should be received from citizens’ mobile phones. We are told that there are systems that work on the basis of aggregate and anonymised information but, in fact, aggregate information about population groups is obtained from information on each individual mobile device and includes people’s location. The problem stems from the fact that this is done centrally and poses risks, such as the information receiver using it for surveillance or commercial purposes. The alternative would be to create a decentralised system that collects information in such a way that each individual manages their data. This system could monitor if any symptoms of the disease are presented and also, thanks to Bluetooth, if the person has been in close proximity to other people with symptoms. Finally, the person voluntarily decides to download their data and incorporate them into the system to monitor the spread of the epidemic.
From health to cities. What is the potential of artificial intelligence to improve life in cities?

There is no doubt that artificial intelligence holds great potential to improve cities. But a precondition is needed: sensors everywhere. We would have to install sensors in the public space to detect forms of behaviour, and again that clashes with rights and freedoms. If, for example, the purpose is to have information on the mobility of both vehicles and pedestrians, sensors that fully respect privacy would suffice. In this case, it would be a question of ruling out computer vision systems that read licence plates and even detect faces.
Mobility, energy and security are the three major areas for implementing AI in cities such as Barcelona. The common denominator of artificial intelligence is to develop algorithms that optimise decisions. In fact, AI, based on data and/or knowledge, endeavours to make better decisions in a flexible and dynamic way. Some examples would be to better regulate traffic lights according to the volume of traffic, to adapt the intensity of buses, to encourage smart parking or to regulate street lighting. And what will radically change mobility in cities in the medium term will be self-driving cars. If this is well managed, efficiency will be increased, energy will be saved and greenhouse gas emissions will be reduced.
Can artificial intelligence improve city governance and strengthen the participation of social stakeholders in decision-making?
Again, the potential exists but there needs to be political will and resources. Multi-agent systems and opinion aggregators could be designed based on arguments and counter-arguments. The AI system could be attached to a simulator that deploys different scenarios depending on the decisions made. It would be a way to make participation more appealing to citizens; participation could even be encouraged through rewards, such as tax rebates to people who actively participate.
What is the state of artificial intelligence research in Catalonia and in Spain?
“We have excellent research groups. We are part of the club of the 15 most competitive AI countries on a global scale.”
We have a huge critical mass. We have excellent research groups that publish in the best journals, both on AI and on computer vision. On a European scale, I’d say that only Britain, Germany, France, the Netherlands and Italy clearly surpass us, and Israel also overtakes us. If we look around the world, there are about five or six more countries that surpass us. We are part of the club of the 15 most competitive AI countries on a global scale.
And what about companies?
We aren’t bad at all. A recent French study places Spain as the fourth European country in terms of the creation of new companies focused on AI. A large number of start-ups are emerging, especially in Catalonia. Hopefully many will be consolidated.
Finally, is artificial intelligence more oriented today towards profit or the common good?
Lately, the big driving force behind AI is, without a doubt, profit. The priority of Big Tech companies is to make a lot of money, even if they say their priority is to develop AI to build a better world. On the other hand, public authorities and academia have a wide range of opportunities to do many things for the common good.
The newsletter
Subscribe to our newsletter to keep up to date with Barcelona Metròpolis' new developments