Artificial intelligence fostering shared and sustainable mobility
The impacts of artificial intelligence
- Dossier
- Jul 23
- 11 mins
AI has the potential to help manage complex processes, such as mobility, in city life. Simulators and servers that match people offering their cars with those who need to get around are conducive to reducing traffic by 80% and cutting CO2 emissions by 70%.
Earlier this spring, one day after lunch, I had to go somewhere in the city of Barcelona to run some errands. The prospect of a long, sunny evening made me plan a bike ride along the seafront (I live near the sea). Since my destination was not very well served by public transport, I decided to take my car; besides, there was a car park nearby and it wasn’t rush hour. This was a mistake. When I returned from my errand, I drove that afternoon to the car park I had chosen. With traffic flowing smoothly, my plans were going reasonably well. But the return journey was a very different story. Within minutes, and for no apparent reason, I found myself stuck in a traffic jam with no way out. Perhaps there had been an accident or there were roads blocked by roadworks or demonstrations. I don’t know. By the time I got home, I had run out of time, but most of all, I didn’t fancy going for that bike ride. The traffic jam had put me in a bad mood, not to mention the petrol I had burned, the time I had wasted and the air and noise pollution I had generated.
This incident serves to illustrate the traffic problems in big cities. Public transport is still the best solution for mobility in cities, but it is a partial solution: there will always be areas that are not adequately served, because when cities grow, it takes time – years – for new neighbourhoods to be provided with public transport links rapidly. This process is often so drawn out that new neighbourhoods emerge in which the problem recurs. The generic solution is inevitably shared mobility; it is a matter of removing vehicles transporting only one passenger from the massive and frenetic human anthill that a big city turns into at rush hour.[1] There are long-standing solutions to which artificial intelligence is now giving fresh impetus.
Ridesharing on a large scale
The concept of ridesharing or carpooling emerged at the same time as vehicles, and their use stretches back decades. There are different versions of this idea. Here we refer to the case in which a driver offers their vehicle to take other passengers for a ride (in specialist literature it is known as peer-to-peer ridesharing). Other forms of ridesharing may even increase the number of vehicles.
Advances in artificial intelligence coupled with communication facilities are making it possible to build the technology needed to implement ridesharing in a big city.
This method has been employed since the second half of the last century in restricted areas, such as work colleagues who share a car to commute to the same destination or members of the same family heading to different parts of the city. In both cases, the communication between the carpoolers is direct, incurring no extra cost, and the optimisation issue to be solved is straightforward. But its large-scale application is still pending. Today, advances in artificial intelligence coupled with communication facilities are making it possible to build the technology needed to implement this approach in a big city. We have developed methods that demonstrate the feasibility of this idea on a large scale, with a simulator that predicts the expected benefits.
The proposed system works in such a way that each person offering a ride or needing a ride sends a request to a server. The server continuously provides a shared mobility service: it receives the offers and requests and, in a limited space of time – one minute –, it combines the offers of rides that have not yet been availed of with the requests that have not yet been served, by means of a stochastic optimisation process.[2] It then allocates the rides offered and requested considered in that cycle, and continues the process. A person generally does not have to wait more than five minutes between sending a request and receiving a response.
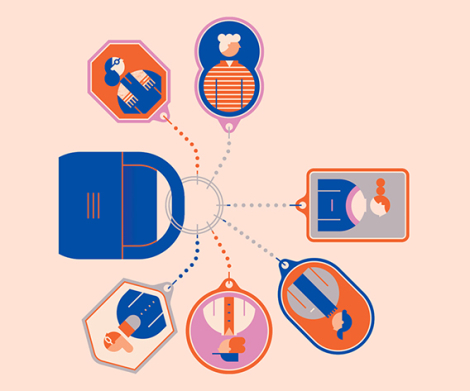
The savings it can provide depend on the criteria prioritised. There are several, and we shall focus on two here, which are handled as metrics: the extra time required versus an individual journey and the adoption ratio (the percentage of vehicles citywide that are carpooling). Based on actual traffic data over two days[3] in New York, a weekday – Thursday 1/12/2016 – and a weekend day – Saturday 3/12/2016 –, our experimental study notes that the reduction in air pollution clearly improves in tandem with the adoption ratio, which is directly correlated with the reduction in the number of journeys and total distance travelled. These data improve as we allow more extra time per shared journey, accepting longer delays, up to ten minutes in the worst case. In round figures, with a 100% adoption ratio, with all seats occupied in the vehicle and the highest environmental priority, a 70% cut in CO2 emissions and an 80% reduction in traffic is achieved. Conversely, in the worst-case scenario, a 20% adoption rate with the lowest environmental priority, which represents an average delay of ten seconds, an 8% cut in CO2 emissions is achieved and traffic is reduced by 15%. Thus, even at low levels of implementation in a big city like New York, the proposed method delivers significant environmental benefits (a 1% reduction in CO2 emissions is equivalent to a saving of 1.5 tonnes of CO2). In intermediate scenarios, the benefits obtained lie between these two extremes.
A simulator to aid decision-making
With a view to providing a flexible system that delivers different results based on the variation of ridesharing priorities, we have developed a simulator that performs the resolution process, using the selected priorities as parameters. This simulator is a valuable tool, as it facilitates the precise quantification of the benefits yielded by the implementation of this idea for a specific city with specific preferences. Furthermore, it acts as a decision-making tool for government authorities that have to decide on urban mobility policies. The simulator must be fed with data from the map of the target city, and is available on the website of the Artificial Intelligence Research Institute (IIIA)[4] for the case analysed.
The simulator offers two methods: simulation and analysis. In the first method, once it provides values for the model’s different parameters, it solves the proposed problem and displays the results for the urban area under consideration. In the second, it performs reverse engineering: it sets the goals to be accomplished in terms of environmental benefits and extra time allowed for ridesharing, and calculates the peer-to-peer ridesharing policy required to achieve them.
If citizens cooperate, shared mobility rollout will help mitigate the severe traffic congestion that often clogs our streets.
The procedure is fast and sustainable as it is executed within a time limit (one minute for the optimisation process, five minutes in total) and does not require special infrastructure or intensive calculation. If citizens cooperate, its rollout will help mitigate the severe traffic congestion that often clogs our streets. It constitutes a viable approach to rethinking urban mobility and a prerequisite for seizing the opportunities that cities offer their inhabitants and for achieving environmental benefits that are urgently needed in the 21st century.
Other applications
We have developed another AI project for sustainable mobility[5] that recommends “green routes” to citizens who move around Barcelona on foot or by bicycle, considering environmental quality (reduced levels of pollution, presence of green areas, etc.) and the aim to minimise traffic time. These recommendations are based on the data available in Open Data BCN[6] and those collected from the network of sensors that monitor the level of pollutant emissions[7] and air quality.[8] Our simulations indicate that these green routes significantly reduce the exposure of pedestrians or cyclists to pollution and noise (from 20% to 40%, while increasing transit time by 10% to 20%), compared to the routes recommended by standard services such as Google Maps.
In addition, an alternative to the usual delivery of goods in cities has been identified, known as co-loading: when several logistics providers share space on the same vehicle to distribute their products. In practical terms, this would mean a reduction in the number of freight vehicles in each neighbourhood, as the same delivery vehicle would distribute the orders of several suppliers. This idea has proven to be very useful in industrial logistics, together with backhauling, which consists of using the return journey of empty trucks to transport other goods. Its application is promising in cities (known as last mile delivery in the transport world).
Artificial intelligence for good (AI for good) could make cities more liveable, increasing the quantity and quality of natural environments, reducing social inequalities and improving access to health and knowledge.
There are many other AI applications to improve mobility in cities: public transport dynamically adapted to user demand, smart traffic lights, parking management, etc. A second stage includes autonomous vehicles and the internet of things. Together, these functionalities define a new concept of smart city, where people’s needs are technology-mediated to satisfy them in an efficient and healthy manner.
The strategies outlined in this article are just a small grain of sand in what is known as artificial intelligence for good (AI for good). Making cities more liveable, increasing the quantity and quality of natural environments, reducing social inequalities and improving access to health and knowledge are some of its fundamental goals. It is these ways to improve the world that give deeper meaning to our technical efforts. They are aligned with the Sustainable Development Goals,[9] laid out by the United Nations in its 2030 Agenda. Artificial intelligence has a big part to play in this initiative.
[1] The head of Barcelona’s Urban Ecology Agency said in an interview: “Sixty-five per cent of the space in capital cities is allocated to parking or to the circulation of traffic, and this means that public places cannot be used for other purposes”. www.eldiario.es/alava/ocupado-espacio-ciudades-aparcar-circular_1_571842...
[2] The resolution also incorporates data from the urban area under consideration, obtained from Google Maps. A heuristic adopts the objective function, which speeds up its optimisation. The complete procedure is outlined in detail in https://ieeexplore.ieee.org/document/8917688.
[3] A separate analysis was conducted for each day and very similar findings were obtained. The exact data from both studies can be found at https://ieeexplore.ieee.org/document/8917688.
[4] https://ridesharing.iiia.csic.es
[5] Calo, S., Bistaffa, F., Jonsson, A. and Gómez, V. “A novel message passing approach to spatial air quality prediction in urban areas”. Deep Learning Barcelona Symposium. 2022.
References
Bistaffa, F., Blum, C., Cerquides, J., Farinelli, A. i Rodríguez-Aguilar, J. A. “A computational approach to quantify the benefits of ridesharing for policy makers and travellers”, IEEE Transactions on Intelligent Transportation Systems, 22(1), 119-130. 2021. http://ow.ly/OLT350OyQqB
De Jonge, D., Bistaffa, F. i Levy, J. “Multi-objective vehicle routing with automated negotiation”. Applied Intelligence, 52, 16916-16939. 2022. http://ow.ly/Lfpl50OyQrc
Diao, M., Kong, H. i Zhao, J. “Impacts of transportation network companies on urban mobility”. Nature Sustainabiliy, 4, 494-500. 2021. http://ow.ly/kZYh50OyQsx
Nikitas, A., Michalakopoulou, K., Njoya, E. T. i Karampatzakis, D. “Artificial intelligence, transport and the smart city: Definitions and dimensions of a new mobility era”. Sustainability, 12(7), 2789. 2020. http://ow.ly/HZJR50OyPKq
Inteligencia artificial Ramon López de Mántaras Badia and Pedro Meseguer González. “¿Qué sabemos de?” Collection, Los Libros de la Catarata, 2017
The newsletter
Subscribe to our newsletter to keep up to date with Barcelona Metròpolis' new developments