Myths and realities of artificial intelligence
The impacts of artificial intelligence
- Dossier
- Jul 23
- 9 mins
The artificial intelligences developed to date are specific to very concrete tasks. However, they do not have the capacity to understand what they actually do, nor can they interact with the environment or generate certain types of knowledge, such as common sense, which is the product of our experiences. While we are speculating whether AI will replace human intelligence, we are getting distracted from the real issues: who is controlling its applications and what is it being used for?
In the 1960s, Arthur Clarke asserted that, “any sufficiently sophisticated technology is indistinguishable from magic”. Artificial intelligence (AI) is a prime example of this. Its capabilities seem to be boundless. Nevertheless, in actual fact, for more than fifty years, AI has been at a standstill over one of the most fundamental issues: how can machines be equipped with commonsense knowledge.
Commonsense knowledge is the product of our experiences. There are tonnes of commonsense knowledge that people use constantly and that help us to understand the world we live in. Although commonsense knowledge is claimed to be already “emerging” in the recent major language models of generative AI, we cannot say for sure, as the complexity and opacity of these models makes it virtually impossible to determine how they work internally. All we know is that they learn linguistic patterns to predict what the next word in a text is. As I see it, I do not believe this technique allows us to equip AI with common sense, no matter how much we increase the complexity (in terms of number of parameters) of these language models. The problem of endowing machines with common sense is too complicated to believe it can be solved by calculating what the next most likely word is. In fact, in late 2018, the Defence Advanced Research Projects Agency (DARPA), the institution that is the largest investor in research programmes in the United States, set up a long-term, multi-year programme that channels billions of dollars into funding research projects on how to endow machines with commonsense knowledge.
Existing deep learning-based systems are unable to differentiate between causes and effects, such as the sunrise being the reason for the rooster’s crowing and not the other way around.
A fascinating line of research into equipping machines with the knowledge to understand the world revolves around mathematical modelling and learning cause-effect relationships. Existing deep learning-based systems can simply learn symmetric mathematical functions, particularly correlations, but they cannot learn asymmetric relationships and are therefore unable to differentiate between causes and effects, such as the sunrise being the reason for the rooster’s crowing and not the other way around.
AI’s state of play
So what is the real state of affairs concerning AI? In reality, we have specific variants of artificial intelligence, in the sense that each one can execute a single task effectively, even though it does not really understand anything it is doing. Let’s focus on one AI technique, known as deep learning, which, over the last ten years, has achieved spectacular results based on its extraordinary capacity to identify statistical patterns by analysing large amounts of data. According to this technique, there is a programme called AlphaFold[1] that is able to predict 3D models of protein structures, i.e. how a protein folds in three-dimensional space. Solving the protein-folding problem is important for discovering and designing new drugs, because the role of proteins depends on their three-dimensional structure. Another very recent successful example of deep learning, combining neural networks and reinforcement learning, is the aforementioned generative AI, which can process natural language as well as music, images or videos from an initial description. Despite their apparent intelligence, these systems are very limited. In fact, they do not learn anything in the human sense of learning, since, unlike us, they do not learn incrementally, they cannot relate what they learn to what they have learned before and they have practically no capacity for generalising or reasoning.
Excessive anthropomorphism is the main reason for society’s misperception of the real state of artificial intelligence.
So why is it widely believed that AI is on the verge of equalling human intelligence? Moreover, based on this premise, why are hypothetical artificial intelligences superior to human intelligence being predicted? In my opinion, excessive anthropomorphism is the main reason for society’s misperception of the real state of artificial intelligence. When we discover the outstanding results achieved by an AI in performing a very specific task, we tend to generalise and attribute this to its capacity to do almost anything we humans do, and even to do it much better. In reality, what today’s AI systems have is not intelligence but “competence without understanding” in the sense that Daniel Dennett describes in his book From Bacteria to Bach and Back: The Evolution of Minds[2]. That is, systems that can become highly skilled at performing specific tasks, such as recognising a series of elements in an image or generating text, but with absolutely no understanding of the nature of these elements or of the properties and connections between them.
The problem is that current AI systems do not have access to real-world reference points that inform the content of words because, as non-corporeal artificial intelligences, they cannot interact with the world. This inability to truly understand the world makes it impossible for major language models such as GPT-4 to understand the meaning of the texts they generate. In fact, the designers of these systems do not care about the absence of real-world reference points. They only care about rhetorical power, fooling listeners and readers into believing that they understand language like humans do, even though they generate a plethora of falsehoods.
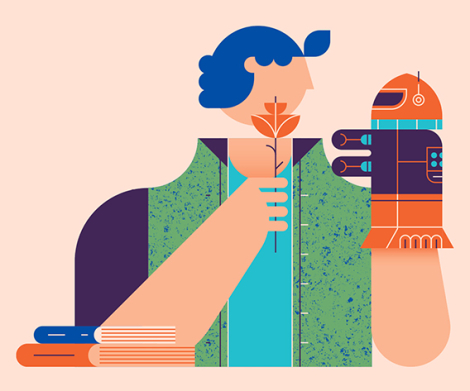
In 2021, researchers Timnit Gebru and Margaret Mitchell, co-leaders of Google’s ethics team at that time, already warned of the risk of people ascribing communicative intent and language understanding to human-like devices.[3] Ironically, because they made this key ethical issue public, Google fired them. Emily M. Bender, an expert in computational linguistics, rightly argues that creating technology that mimics humans by posing as something human demands clarity about what it means to be human, otherwise we run the risk of dehumanising ourselves.
Others, such as Daniel Dennett, are even more convincing. Dennett argues that we cannot live in a world with counterfeit people, because a society with counterfeit people who we cannot tell apart from real people would no longer be a society. He reminds us that making counterfeit money is a criminal act and says that counterfeiting people is just as serious, if not more so. Furthermore, artificial people cannot be held accountable, and that makes them amoral actors, with a tremendous capacity to generate multiple falsehoods, i.e. with the capacity to misinform. Dennett adds that it is the creators of these technologies who are to blame. If they do not start being regulated, democracy may soon be ridden with misinformation and the resulting polarisation. The stability and security of society are at stake.
Towards more generic artificial intelligence
The most challenging tasks to perform are those that demand interaction with dynamic, uncertain, unpredictable and unconstrained environments. Designing systems that have these capabilities calls for integrating developments from many domains of AI. In particular, we need knowledge representation languages that encode information about many different types of objects, situations, actions, etc., as well as their properties and the connections between them, especially cause-effect relationships, in order for artificial intelligence to understand the world. We also need new algorithms that, based on these representations, can robustly and efficiently solve problems on practically any subject. Finally, given that they will need to acquire an almost unlimited amount of knowledge, these systems will have to be able to learn continuously throughout their existence. In short, it is imperative that cognitive architectures properly incorporate perception, representation, reasoning, action and learning. This is a major problem, as we do not yet know how to integrate all these elements of intelligence. Addressing this issue is a first step towards generic artificial intelligence.
Final reflections
Human intelligence is the main yardstick for achieving the ultimate goal of generic AI, but no matter how sophisticated this future AI becomes, it will always differ from human intelligence. This is because the mental development required for complex intelligence depends on interactions with the environment, and these in turn depend on the body, in particular the sensory and motor systems. They constitute systems that do not exist in the case of non-corporeal artificial intelligence and are very different from ours in the case of corporeal artificial intelligence.
The danger of AI is not the supposed technological singularity resulting from hypothetical future artificial superintelligence. Apocalyptic discourses concerning the long-term dangers may have been brought to the table in a self-serving fashion, in order to distract us from the real problems it presents today: privacy, manipulation, the mass surveillance and control of citizens, the autonomy of systems (in particular autonomous weapons), overconfidence in AI capabilities, the bias of algorithms, unaccountability in justifying their decisions, and the excessive power of a few big technology companies.
We need future citizens who are more and better informed, better able to assess technological risks, citizens with a more critical mind and capable of asserting their rights.
Broadly speaking, the more sophisticated AI systems become, the more accountability we should demand from their designers and programmers, to ensure their compliance with legal and ethical principles. Artificial intelligence does not, and never will, have intentionality; they are a reflection of the intentions and biases of the teams of programmers and agencies involved in their implementation and deployment. We are the moral agents, not machines. This is why many experts point to the need to regulate their development and even to prohibit their use in cases of extreme risk, such as lethal weapons. But besides regulation, citizens, especially politicians, need to be educated about the benefits and risks of smart technologies. We need future citizens who are more and better informed, better able to assess technological risks, citizens with a more critical mind and capable of asserting their rights. This education process must start in schools and continue at university. In particular, science and engineering students need to be trained in ethics to better understand the social implications of the technologies they will develop. It is only by investing in education that will we achieve a society that can reap the benefits of smart technologies while minimising their risks. Artificial intelligence will thus play a major role in driving the progress of humankind.
[1] Jumper, J., Evans, R., Pritzel, A. et al. “Highly accurate protein structure prediction with AlphaFold”. Nature, 596, 583-589. 2021. http://ow.ly/XiVW50OnOjm
[2] Dennet, D. C. From Bacteria to Bach and Back: The Evolution of Minds. Penguin Books, 2017.
[3] Bender, E. M., Gebru, T., McMillan-Major, A. i Shmitchell, S. “On the Danger of Stochastic Parrots: Can Language Models Be Too Big?”. ACM Conference on Fairness, Accountability, and Transparency, 610-623. 2021.
Books
Inteligencia artificial Ramon López de Mántaras Badia and Pedro Meseguer González. “¿Qué sabemos de?”, Collection, Los Libros de la Catarata, 2017
The newsletter
Subscribe to our newsletter to keep up to date with Barcelona Metròpolis' new developments